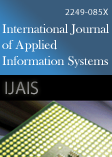
- P. Sasikala and V. Vidhya 2012. An Efficient Concept-based Mining Model for Deriving User Profiles. International Journal of Applied Information Systems. 1, 6 (February 2012), 26-34. DOI=http://dx.doi.org/10.5120/ijais450187
-
@article{10.5120/ijais2017451568, author = {P. Sasikala and V. Vidhya }, title = {An Efficient Concept-based Mining Model for Deriving User Profiles}, journal = {International Journal of Applied Information Systems}, issue_date = {February 2012}, volume = {1}, number = {}, month = {February}, year = {2012}, issn = {}, pages = {26-34}, numpages = {}, url = {/archives/volume1/number6/99-0187}, doi = { 10.5120/ijais12-450187}, publisher = { xA9 2010 by IJAIS Journal}, address = {} }
-
%1 450187 %A P. Sasikala %A V. Vidhya %T An Efficient Concept-based Mining Model for Deriving User Profiles %J International Journal of Applied Information Systems %@ %V 1 %N %P 26-34 %D 2012 %I xA9 2010 by IJAIS Journal
Abstract
Software testing is an important activity in the software development life cycle and it is widely used validation approach in software industry, deployed by programmers and testers. The program with the moderate complexity cannot be tested completely. Innovative methods are needed to perform testing as a whole and unit testing in particular with minimum effort and time. Unit testing is mostly done by developers under a lot of schedule pressure since the software companies find a compromise among functionality, time to market and quality. Thus there is a need for reducing unit testing time by optimizing and automating the process. Test suite generation is an error-prone, tedious and time consuming part of unit testing. Two techniques are proposed to automatically generate test cases from the input domain using scatter search and tabu search for branch coverage criteria with respect to cyclomatic complexity measure.
References
- E. Agichtein, E. Brill, and S. Dumais, “Improving Web Search Ranking by Incorporating User Behavior Information,” Proc. ACM SIGIR, 2006.
- E. Agichtein, E. Brill, S. Dumais, and R. Ragno, “Learning User Interaction Models for Predicting Web Search Result Preferences,”Proc. ACM SIGIR, 2006.
- R. Baeza-yates, C. Hurtado, and M. Mendoza, “Query Recom-mendation Using Query Logs in Search Engines,” Proc. Int’l Workshop Current Trends in Database Technology, pp. 588-596, 2004.
- D. Beeferman and A. Berger, “Agglomerative Clustering of a Search Engine Query Log,” Proc. ACM SIGKDD, 2000.
- K.W. Church, W. Gale, P. Hanks, and D. Hindle, “Using Statistics in Lexical Analysis,” Lexical Acquisition: E xploiting On-Line Resources to Build a Lexicon, Lawrence Erlbaum, 1991.
- Z. Dou, R. Song, and J.R. Wen, “A Largescale Evaluation and Analysis of Personalized Search Strategies,” Proc. World Wide Web (WWW) Conf., 2007.
- S. Gauch, J. Chaffee, and A. Pretschner, “Ontology-Based Personalized Search and Browsing,” ACM Web Intelligence and Agent System, vol. 1, nos. 3/4, pp. 219-234, 2003.
- T. Joachims, “Optimizing Search Engines Using Clickthrough Data,” Proc. ACM SIGKDD, 2002.
- K.W.T. Leung, W. Ng, and D.L. Lee, “Personalized Concept-Based Clustering of Search Engine Queries,” IEEE Trans. Knowl-edge and Data Eng., vol. 20, no. 11, pp. 1505-1518, Nov. 2008.
- B. Liu, W.S. Lee, P.S. Yu, and X. Li, “Partially Supervised Classification of Text Documents,” Pr o c. I nt ’ l C on f . M a ch in e Learning (ICML), 2002.
- F. Liu, C. Yu, and W. Meng, “Personalized Web Search by Mapping User Queries to Categories,” Proc. Int’l Conf. Information and Knowledge Management (CIKM), 2002.
- W. Ng, L. Deng, and D.L. Lee, “Mining User Preference Using Spy Voting for Search Engine Personalization,” ACM Trans. Internet Technology, vol. 7, no. 4, article 19, 2007.
- M. Speretta and S. Gauch, “Personalized Search Based on User Search Histories,” Proc. IEEE/WIC/ACM Int’l Conf. Web Intelligence,2005.
- Q. Tan, X. Chai, W. Ng, and D. Lee, “Applying Co-training to Clickthrough Data for Search Engine Adaptation,” Proc. Database Systems for Advanced Applications (DASFAA) Conf., 2004.
- J.R. Wen, J.Y. Nie, and H.J. Zhang, “Query Clustering Using User Logs,” ACM Trans. Information Systems, vol. 20, no. 1, pp. 59-81, 2002.
- Y. Xu, K. Wang, B. Zhang, and Z. Chen, “Privacy-Enhancing Personalized Web Search,” Proc. World Wide Web (WWW) Conf., 2007.
- K.Wai, T.Leung, D.L.Lee,”Deriving Concept-based user profiles from search engine logs” ,IEEE transactions on Knowledge and data engineering”, Vol.22, No.2,July 2010
- Kai Yu, Xiaowei Xu,Hans-Peter Kriegel,”Probabilistic memory-based Collaborative Filtering”, IEEE transactions on Knowledge and Data Engineering.
Keywords
Clustering, Collaborative Filtering, Personalization, Query formulation, User profiles, Personality diagnosis