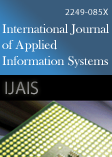
- S. Vijayasankari and K. Ramar 2012. Common Hybrid Feature Selection for Modeling Intrusion Detection System and Cyber Attack Detection System. International Journal of Applied Information Systems. 3, 6 (July 2012), 16-22. DOI=http://dx.doi.org/10.5120/ijais450550
-
@article{10.5120/ijais2017451568, author = {S. Vijayasankari and K. Ramar}, title = {Common Hybrid Feature Selection for Modeling Intrusion Detection System and Cyber Attack Detection System}, journal = {International Journal of Applied Information Systems}, issue_date = {July 2012}, volume = {3}, number = {}, month = {July}, year = {2012}, issn = {}, pages = {16-22}, numpages = {}, url = {/archives/volume3/number6/236-0550}, doi = { 10.5120/ijais12-450550}, publisher = { xA9 2010 by IJAIS Journal}, address = {} }
-
%1 450550 %A S. Vijayasankari %A K. Ramar %T Common Hybrid Feature Selection for Modeling Intrusion Detection System and Cyber Attack Detection System %J International Journal of Applied Information Systems %@ %V 3 %N %P 16-22 %D 2012 %I xA9 2010 by IJAIS Journal
Abstract
Intrusion Detection Systems (IDS) and Cyber Attack Detection System (CADS) have to be provided in a Generalised Discriminant Analysis Algorithm. It is an important approach to nonlinear features and extensively used tool for ensuring network security. Complex relationships exist between the features, which are difficult for humans to discover. The conventional Linear Discriminant Analysis feature reduction technique is not suitable for nonlinear data set. Artificial Neural Network and C4. 5 classifiers to result in supervisory algorithm are used. If real-time detection is desired IDS must reduce the amount of data to be processed. Currently IDS examine all data features to detect intrusion or misuse patterns. Some of the features may be redundant or contribute little to the detection process. The purpose of this research work is to identify important input features in building IDS that is computationally efficient and effective. The performance of two feature selection algorithms involving Bayesian Networks (BN) and Classification and Regression Trees (CART) and an ensemble of BN and CART were investigated. Empirical results indicate that significant input feature selection is important to design IDS with efficient, effective and lightweight for real world detection systems. Finally, hybrid architecture for combining different feature selection algorithms for real world intrusion detection was proposed.
References
- American computer Emergency response Team / Coordination centre (CERT), http://www. cert. org. , January, 2012.
- Kemal Polat, Salih GxFCne?, and Ahmet Arslan 2008, 'A cascade learning system for classification of diabetes disease: Generalized Discriminant Analysis and Least Square Support Vector Machine', Expert Systems with Applications, Vol. 34, pp. 482-487.
- Jing Gao, Haibin Cheng and Pang-ning Tan, 2006 'A novel framework for Incorporating Labeled Example into anomaly detection', Proceedings of the Siam Conference on Data mining
- Gopi K, Kuchimanchi, Vir V Phoha, Kiran S Balagani, Shekhar R Gaddam 2004, 'Dimension Reduction Using Feature Extraction Methods for real-time Misuse Detection Systems', Proceedings of the IEEE on Information.
- Mukkamala S, Sung A. H. and Abraham A. 2003, Intrusion Detection Using Ensemble of Soft Computing Paradigms, Third International Conference on Intelligent Systems Design and Applications, Springer Verlag Germany, pp. 239-248.
- MIT Lincoln Laboratory. http://www. ll. mit. edu/IST/ideval/
- Information security report, http://www. isecu-tech. com. tw/. 2012.
- KDDCup99dataset, August 2003 http://kdd. ics. uci. edu/databases/kddcup99/kddcup99. html
- Brieman L. , Friedman J. , Olshen R. and Stone C. 1984, Classification of Regression Trees. Wadsworth Inc.
- Lee W. , Stolfo S. and Mok K. 1999, A Data Mining Framework for Building Intrusion Detection Models, In Proceedings of the IEEE Symposium on Security and Privacy.
- Nallusamy. R, Jayarajan. K, Duraiswamy. K. 2009, 'Intrusion Detection In Mobile Ad Hoc Networks Using GA Based Feature Selection', Georgian Electronic Scientific Journal: Computer Science and Telecommunications, No. 5 (22), pp. 28-35.
- Chebrolu, S. , A. Abraham, J. Thomas. 2005 , 'Feature deduction and ensemble design of intrusion detection systems', Journal of Computers & Security, Vol-24, pp. 295-307.
- Khoshgoftaar T. M, Nath S. V, Zhong S, and Seliya. N. 2005, "Intrusion Detection in Wireless Networks Using Clustering Techniques with Expert Analysis," Proc. Fourth Int'l Conf. Machine Learning and Applications.
- A. Boukerche, K. R. L. Juc, J. B. Sobral, and M. S. M. A. Notare. 2004, "An Artificial Immune Based Intrusion Detection Model for Computer and Telecommunication Systems," Parallel Computing, Vol. 30, pp. 629-646.
- Y. -H. Liu, D. -X. Tian, and D. Wei. 2006, 'A Wireless Intrusion Detection Method Based on Neural Network', Proc. Second IASTED Int'l Conf. Advances in Computer Science and Technology.
- W. Zhao, R. Chellappa, and N. Nandhakumar. 1998, "Empirical Performance Analysis of Linear Discriminant Classifiers," Proc. Computer Vision and Pattern Recognition, pp. 164-169.
- Baudt. G and Anouar. F. 2000, 'Generalized Discriminant Analysis Using a Kernal Approach,' Neural Computation.
- Fukunaga. K. 1990, 'Introduction to Statistical Pattern Classification', Academic Press, San Diego, California, USA.
- Quinlan, J. R. 1994, 'C4. 5: Programs for Machine Learning', Machine learning, Vol. 16, pp. 235-240.
- Shailendra Singh and Sanjay Silakari. 2009, 'Generalized Discriminant Analysis algorithm for feature reduction in Cyber Attack Detection System', International Journal of Computer Science and Information Security, Vol. 6, pp. 173-180.
Keywords
Cyber attack, Data mining, Hybrid feature selection, Intrusion detection, Classification