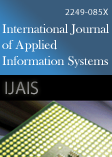
- F. A. Mazarbhuiya and Yusuf Perwej 2015. An Efficient Method for Generating Local Association Rules. International Journal of Applied Information Systems. 9, 2 (June 2015), 1-5. DOI=http://dx.doi.org/10.5120/ijais451368
-
@article{10.5120/ijais2017451568, author = {F. A. Mazarbhuiya and Yusuf Perwej}, title = {An Efficient Method for Generating Local Association Rules}, journal = {International Journal of Applied Information Systems}, issue_date = {June 2015}, volume = {9}, number = {}, month = {June}, year = {2015}, issn = {}, pages = {1-5}, numpages = {}, url = {/archives/volume9/number2/753-1368}, doi = { 10.5120/ijais15-451368}, publisher = { xA9 2015 by IJAIS Journal}, address = {} }
-
%1 451368 %A F. A. Mazarbhuiya %A Yusuf Perwej %T An Efficient Method for Generating Local Association Rules %J International Journal of Applied Information Systems %@ %V 9 %N %P 1-5 %D 2015 %I xA9 2015 by IJAIS Journal
Abstract
Carving association rules from any available set is a pre-defined problem and there are a variety of methods available for the extraction of association rules. Almost in all the cases the major emphasis is given to the generating most occurring itemsets rather than the extraction of association rules. Only a few numbers of researchers have through some light on this problem. Extracting generally most occurring itemsets for sometimes does not guarantee that particular dataset will occur in its lifetime. . For finding local association rules of the form A Þ X - A, where X and A are itemsets that hold in the interval [t, t¢] and . In order to calculate the confidence of the rule A Þ X – A in the interval [t, t¢], it is required to know the supports of both X and A in the same interval [t, t¢]. The supports of X and any of its subset A may not be available for the same time interval - A may be frequent in an interval greater than [t, t¢]. So, they have loosely defined local association rule as confidence in the rule A Þ X – A can't be calculated in interval [t, t¢]. In this paper, we present a latest approach for finding association rules from generally most occurring item sets using Rough Set and Boolean reasoning. The rules carved are called local association rules. The efficacy of the proposed approach is established through experiment over retail dataset that contains retail market basket data from an anonymous Belgian retail store.
References
- Agrawal, R. , Imielinski, T. , and Swami, A. N. ; Mining association rules between sets of items in large databases, In Proc. of 1993 ACM SIGMOD Int'l Conf on Management of Data, Vol. 22(2) of SIGMOD Records, ACM Press, (1993), pp 207-216.
- Agrawal, R. , and Srikant, R. ; Fast Algorithms for Mining Association Rules, In Proc. of the 20th VLDB Conf. , Santiago, Chile, 1994.
- Ale, J. M. , and Rossi, G. H. ; An Approach to Discovering Temporal Association Rules, In Proc. of 2000 ACM symposium on Applied Computing (2000).
- Antunes, C. M. , and Oliviera, A. L. ; Temporal Data Mining an overview, Workshop on Temporal Data Mining-7th ACM SIGKDD Int'l Conf. on Knowledge Discovery and Data Mining, (2001).
- Chen, X. and Petrounias, I. ; A framework for Temporal Data Mining; Proceedins of the 9th International Conference on Databases and Expert Systems Applications, DEXA '98, Vienna, Austria. Springer-Verlag, Berlin; Lecture Notes in Computer Science 1460 (1998) 796-805.
- Chen, X. and Petrounias, I. ; Language support for Temporal Data Mining; In Proceedings of 2nd European Symposium on Principles of Data Mining and Knowledge Discovery, PKDD '98, Springer Verlag, Berlin (1998) 282-290.
- Chen, X. , Petrounias, I. , and Healthfield, H. ; Discovering temporal Association rules in temporal databases; In Proceedings of IADT'98 (International Workshop on Issues and Applications of Database Technology (1998) 312-319.
- Mahanta, A. K. , Mazarbhuiya, F. A. , and Baruah, H. K. ; Finding Locally and Periodically Frequent Sets and Periodic Association Rules, In Proc. of 1st Int'l Conf. on Pattern Recognition and Machine Intelligence, LNCS 3776 (2005), pp. 576-582.
- Manilla, H. , Toivonen, H. and Verkamo, I. ; Discovery of frequent episodes in event sequences, Data Mining and Knowledge Discovery: An International Journal 1(3), (1997). pp. 259-289.
- Motwani R. , Cohen, E. , Datar, M. , Fujiware, S. , Gionis, A. , Indyk, P. , Ullman, J. D. , and Yang, C. ; Finding interesting association rules without support pruning, In Proceedings of the16th International Conference on Data Engineering (ICDE), IEEE (2000).
- Ramaswamy, S. , Mahajan, S. and Silberschatz, A. ; On the discovery of interesting patterns in association rules, In Proc. of 1998 Int'l Conf. on Very Large Databases, (1998), pp. 368-379.
- Roddick, J. F. , and Spillopoulou, M. ; A Biblography of Temporal, Spatial and Spatio-Temporal Data Mining Research, ACM SIGKDD, (June'1999).
- Paulak, Z. "Rough Sets In Theoretical Aspects of Reasoning about Data", Kluwer, Netherland, 1991.
- Nguyen, H. S. and Nguyen S. H. ; Rough Sets and Association Rule Generation, Fudamenta Informaticae 34 (1999), 1-23.
- Mazarbhuiya F. A. , Mahanta A. K. and Baruah H. K. ; Mining Temporal Patterns in Datasets, Ph. D. thesis, Gauhati University, India, 2007.
- Nguyen H. S. , Slezak D. ; Approximate Reducts and Association Rules- Correspondence and Complexity Results. Proc. Of 7th Int'l Workshops on Rough sets, Fuzzy sets and Granular Soft Computing (RSFDGrC'96), Yamaguchi, Janan, 1996.
- Skowron A. , Rauszer C. ; The dicernibility matrices and functions in information systems, In: R. Slowinski (ed. ): Intelligent Decision support, Handbook of Applications and Advances of the Rough Sets Theory, Kluwer, Dordrecht, pp. 331-362, 1992.
- Wrbewski J. ; Covering with reducts- a fast algorithm for rule generation; Proc. of RSCTC'98, Warsaw, Poland, 1998.
Keywords
Data Mining, Temporal Data Mining, Local Association Rule Mining, Rough Set, Boolean Reasoning